Why Segment?
A common challenge in customer segmentation analysis is to differentiate customers based on features that are relevant to purchasing behavior. For example, clothing retailers may be interested in segmentation based on gender, as this typically relates to style and sizing preferences. However, a financial institution may be more interested in prospects’ age, as age may matter much more than gender when it comes to investment product preferences. In these instances, the business may already know which customer characteristics impact product choices.
In other situations, however, it may be less obvious. For example, imagine that a car dealership is interested in a targeted marketing campaign. Although the dealership probably knows not to advertise minivans to single adults without kids, the dealer may not be able to specify a precise set of customer features that correlate with minivan purchases beyond a vague idea of “people with young children.” It is also possible that the same customer is likely to buy multiple types of cars. For example, a family that just wants a safe car with room for the kids may be indifferent between minivans, station wagons and SUVs. This complicates things.
Applying Machine Learning to Customer Segmentation
One way to create effective a customer segmentation analysis in these more ambiguous cases is to use a clustering algorithm with both customer and product characteristics as inputs. Clustering is a common unsupervised machine learning technique that finds patterns in data, grouping similar observations together. There are many different clustering algorithms, but all translate similarity on a set of features into a single “distance” metric between observations. Starting with a matrix of distances between all pairs of observations, the algorithm will partition the data into groups such that observations within a group are more similar to each other and observations in different groups are less similar to each other.
Returning to the car dealership example, let’s say that the dealer has a large set of transaction records where each transaction contains information about the product purchased, such as the car type, make, model, color and price. Imagine that sales reps at the dealership have diligently entered customer information into a CRM system that integrates with the purchase data. Therefore, for each transaction record in the system, there are also details about the customer, such as his or her age, marital status and whether or not he or she has kids.
Using this integrated transaction-level data, one might use a k-means or k-medoids algorithm to cluster both customers and their purchased products together. This will yield a set of clusters of customers that are similar on characteristics that relate to their shared preference for a specific type of product. For example, there may be a cluster of sports car transactions purchased by middle-aged married men, and another cluster of young, single men and women that purchased small hybrids. By using the transaction records to create segments, the customers will not be segmented based on random shared characteristics, but rather, on the shared characteristics that also correlate with a similar type of purchase.
Transitioning from transaction segments to customer segments
Now, at the end of the day, the dealership wants a set of customer segments, not transaction segments, so how do we get from transaction-based clusters to customer segments without purchase information? One strategy is to subset the customer features out of the larger transaction dataset used in the first round of clustering, and combine those transaction clusters that contain very similar customers. In other words, we might want to combine all of the “young family” transaction segments into one customer group, even if they originally started in two separate transaction clusters because some purchased minivans and others purchased station wagons. For future marketing efforts, all of these customers would receive similar messaging based on one or both of these product types.
A quantitative way to determine which transaction clusters to combine is to calculate a new distance matrix on just the customer features between the centroids (k-means) or medoids (k-medoids) calculated by the transaction clustering process (a centroid is a computed cluster center, whereas a medoid is an actual observation closest to the center of the cluster). These centroids or medoids are the centers of the cluster definitions by the clustering algorithm; all other observations are in a particular cluster because they are closer to the selected cluster’s center than to any of the other clusters’ centers.
If two centroids/medoids were different when using the product features to calculate distance, but are actually very similar when compared on just customer attributes, then the distance between the pair using just the subset of customer attributes would be very small. When evaluating the customer segmentation analysis, one can then set a distance threshold to use in determining which clusters should be combined. After making these combination decisions, any customers in the transaction dataset that were originally assigned to a cluster that no longer exists would be reassigned to the new, combined cluster. Finally, one can generate and evaluate descriptive statistics on the set of customers in each segment to come up with qualitative descriptions of each customer segment for future use.
Conclusion
While this process may ultimately yield customer segments just like any other customer segmentation analysis method that exclusively uses customer characteristics, the iterative approach has benefits. Because the customer segments are constrained to at most the number of combined customer-product transaction clusters, there will only be as many customer segments as there are distinct types of buyers. Buyers that look different on paper but don’t prefer different products will not receive different marketing material, while customers that look similar but have different preferences will receive different marketing. As a result, the approach generates intelligent segmentation—customers are differentiated only to the extent necessary for effective preference-based marketing. And a side benefit is that product clusters can similarly be combined to generate more meaningful product segments in terms of customer choice.
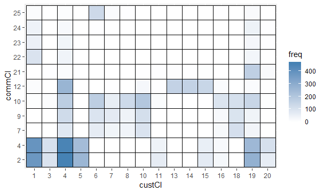
0 Comments