Summary
The Energy industry holds multiple predictive analytics and data science opportunities. One large utility hired a management consulting firm to study their data process. The management consultants identified over $300 Million of value by utilizing the data this utility had already collected over many years.
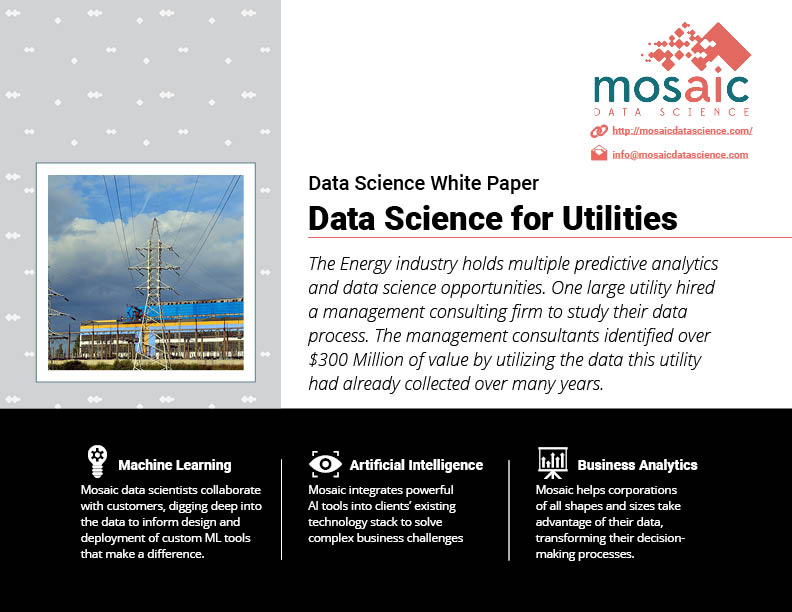
Take Our Content to Go
Identifying Data Science Opportunities in the Utility Sectors
The Energy industry holds multiple predictive analytics and data science opportunities. One large utility hired a management consulting firm to study their data process. The management consultants identified over $300 Million of value by utilizing the data this utility had already collected over many years. With the rise of the Internet of Things (IoT) and data collection technologies becoming more accessible, utility companies have a wealth of data to mine in attacking data science for utilities opportunities. Companies can use predictive analysis and optimization algorithms on these data sets to apply data-driven guidance and decision making to improve efficiency and quality, and reduce costs.
Utilities can run Proof of Concept (PoC) projects to prove value and garner larger investment before spending multiple millions of dollars on a full blown analytics infrastructure. Many companies turn to an expert analytics consulting firm like Mosaic to assist in defining a specific use case, developing a PoC plan and executing on that plan. We work collaboratively with the utility to understand the landscape and bring maximum value. Documents generated from a PoC typically include narrative sections, explaining best practices and processes for various issues, lists of guidelines, checklists for important items to consider each time working on a model, and other instructive elements identified through discussions concerning analytical and business needs.
Mosaic, a leading machine learning consulting firm, has had the pleasure of working with numerous members of North America’s largest utilities. Our data scientists have identified two PoC use cases that are frequently overlooked by most of the big players in the Energy market when it comes to data science for utilities: Maintenance and Customer Voice Analysis.
Predictive Maintenance
Current maintenance paradigms fall into two primary categories:
- Preventive maintenance, which is typically performed according to a fixed schedule and
- Reactive maintenance, which is performed after a failure or drop in performance is observed. This unscheduled maintenance translates to higher maintenance costs, greater downtime and opportunity cost, and increased customer dissatisfaction.
The ideal approach to move maintenance from reactive to proactive is a predictive model, in which equipment sensors and advanced statistical models are used to predict the likelihood of failure, based on current and historical conditions and usage, and to plan the optimal time for maintenance to be conducted. Fortunately, as the cost of sensors and data storage has decreased a wealth of real-time equipment and line data has become available.
As an organization begins to build out a predictive maintenance capability, monetary benefits that this solution offers will be realized. . If a company can predict the likelihood of mechanical asset failure, they can optimize maintenance activities with scheduled, as opposed to unscheduled, maintenance. Organizations can ensure increased equipment availability, effectiveness and run time predictability through understanding historical, current and predicted replacement part availability and replenishment requirements. With greater visibility into equipment health, companies can extend preventive maintenance cycles. Users can start monitoring equipment performance leveraging real time sensor data to accurately predict run time failures and take action to prevent them, as well as identifying maintenance needs that cannot be completed during planned downtime periods. Data-driven diagnostics shorten downtime by ensuring that the right skills, parts, and tools are part of the maintenance response the first time around. Mosaic’s predictive real-time capabilities allow for businesses to start predicting mechanical degradation and failures in time to be prepared – stage inventory, schedule maintenance, and deploy field engineers. The substantiated ROI is measurable and achievable.
A proof of concept use case would involve the following steps:
- Identification of available data regarding production lines and equipment, including historical maintenance and failures, as well as a gap analysis to identify desirable data that is currently unavailable (unstructured data such as maintenance logs can be included using text analytics).
- Decision analysis to determine the maintenance decisions that can be changed based on probability of failure and the operational influences of those decisions.
- Statistical mapping of possible maintenance decisions and outcomes to the available historical data.
- Model development and testing against real assets
- Design of the to-be deployment environment for the model (e.g., integration into an overall operations management dashboard, business process change, etc.)
Figure 1 shows streams of sensor data for an engine and how certain data streams are indicative of an impending failure.
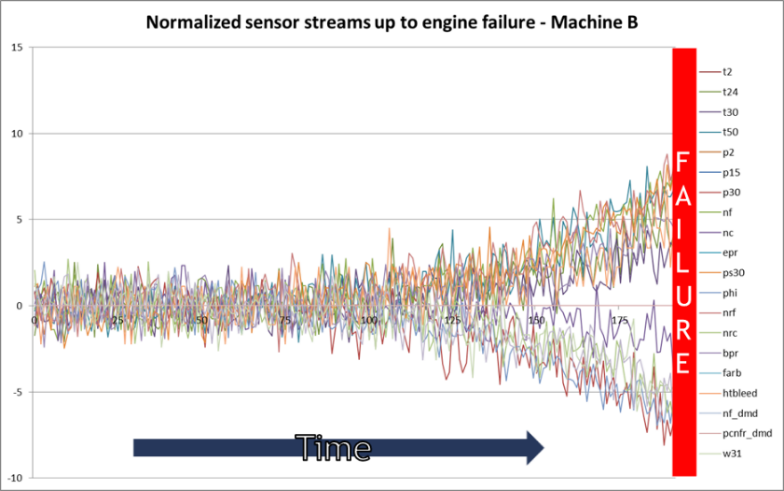
Customer Voice Prediction
In today’s economy, ‘data is king.’ But how can data be king if you can’t make sense of it? To stay ahead of your competition and provide the best value to your customers you need high-quality information – not just data. The IoT, Social Web, and Customer Feedback logs are some of the best sources of data about your customers, but the unstructured text from these raw data sources has to be converted into actionable information using machine learning and natural language processing algorithms.
Mosaic brings the capability to take that unstructured text or voice data and feed it into predictive models to influence and/or predict consumer behavior. Being able to predict behaviors, and/or understand sentiment (how the customer is viewing or feeling about your brand) is a powerful way to enable and optimize decision making and improve the customer experience over time. The customer has a voice that can provide valuable information to facilitate improvement. But if the utility is not hearing that voice, it will be the cause of lost time, revenue and opportunity for growth.
Key example applications include:
- Automated processing of user feedback submissions – routing to the group that can address issues
- Social web comment processing – get out in front of potential problems
- Online market analysis – understand what issues are trending in the market
- Matching help desk tickets to appropriate agent to solve a specific issue
- Categorization of web pages according to topic
- Identification of a referenced product or service